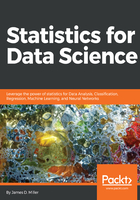
Contextual data issues
A lot of the previously mentioned data issues can be automatically detected and even corrected. The issues may have been originally caused by user entry errors, by corruption in transmission or storage, or by different definitions or understandings of similar entities in different data sources. In data science, there is more to think about.
During data cleaning, a data scientist will attempt to identify patterns within the data, based on a hypothesis or assumption about the context of the data and its intended purpose. In other words, any data that the data scientist determines to be either obviously disconnected with the assumption or objective of the data or obviously inaccurate will then be addressed. This process is reliant upon the data scientist's judgment and his or her ability to determine which points are valid and which are not.